A narrative review of survival normalization in non-Hodgkin lymphoma: useful for better patient counselling and an endpoint for clinical trials?
Background
Overall survival (OS) is considered the gold standard and the most definitive endpoint for measuring cancer outcomes, with non-Hodgkin lymphomas (NHLs) being no exception to this dogma. OS is unaffected by different follow-up routines and clinical investigations for disease progression as well as subjective interpretation of disease status based on these investigations—typical biases inherent to endpoints like progression-free survival (PFS). However, OS is not perfect as it also measures the efficacy of interventions and treatment decisions subsequent to progression, which can lead to systematic bias if the intervention of interest influences these later decisions. In contrast to the majority of solid cancers, NHLs, such as follicular lymphoma (FL) and diffuse large B-cell lymphoma (DLBCL), are typically very chemosensitive diseases and long-term survival is achieved in a substantial fraction of the patients. In DLBCL, 60–70% of the patients achieve durable remissions after first-line therapy with R-CHOP (rituximab, cyclophosphamide, doxorubicin, vincristine, and prednisone), and will be without need of further lymphoma therapy during the rest of their lifetime (1). The remaining 30–40% are either refractory to first-line treatment, relapse after initial response, or fail to complete first-line therapy due to toxicity leading to dismal outcomes (2,3). Non-localized indolent NHLs such as FL or Waldenström’s macroglobulinemia (WM) are incurable with conventional therapies, but long remissions between treatments are common and approximately 80% of immunochemotherapy-treated FL patients are projected to be alive 10 years after diagnosis (4-6). Thus, indolent NHLs are often chronic diseases with long disease-free periods between treatments, although remissions tend to become shorter with each new therapeutic intervention (7,8). The differences in the natural history of aggressive and indolent NHLs have implications for selection of endpoints in clinical trials, which should reflect the balance between expediting clinical trials benefitting patients with unmet medical needs and protecting patient safety, while ensuring that the measured benefit of new therapies translates into relevant outcome improvements for patients. Surrogate endpoints (SEPs) that allow faster readout of study results are of increasing interest in cancer research and time points from which the patient survival becomes identical to that of a background population are among the potential SEPs in NHL trials. Furthermore, such time points are valuable for patient counselling and designing rational survivorship care. In this narrative review, the concept of deriving landmark time points from which no excess mortality exists for NHL patients is reviewed from a statistical and clinical perspective. We present the following article in accordance with the Narrative Review reporting checklist (available at http://dx.doi.org/10.21037/aol-20-4).
Normalization of survival
True cure in the sense of never experiencing relapse throughout the remaining lifetime of a patient can only be concluded after lifelong follow-up, and this individual-level definition of cure is therefore not relevant for SEP purposes. Instead, the definition of cure can be based on a quantification of the residual life expectancy of the patients (9). At diagnosis, the mortality of NHL patients is typically higher than for a matched background population due to the risk of dying from treatment refractory disease, relapse, or treatment complications. However, as time elapses, the patient survival may gradually approach that of similarly aged individuals in the background population as the risk of the aforementioned events decrease. Patients could be considered cured if they reach the time point where the mortality risk for the patient population becomes identical to that of a matched background population. As this population-level definition of cure only applies on a grouped patient level, and therefore does not guarantee freedom of later disease relapses on the individual-level, it has often been called “statistical cure” (10). For the remainder of this review we will use the term “survival normalization”. Two main indicators are commonly considered for survival normalization: (I) the time to survival normalization (TTSN), defined as the time point from which the residual life expectancy among the patients becomes similar to that of the matched background population, and (II) the proportion of the patients who are still alive when survival normalization is reached, termed “the cure fraction” (9). The TTSN is the time point from which patients, in this case NHL patients, face similar risk of death as individuals without NHL. A statistical quantification of the difference between the patient and background population mortality is required to determine the TTSN. Standardized mortality ratios (SMRs), i.e., the fraction of observed number of deaths to the expected number of deaths (in the matched background population) and the loss of expected lifetime (LEL) are frequently used for this purpose (11,12). The cure fraction and 5-year relative survival have also been applied (9,13-15). The advantages and disadvantages of different measures are discussed in detail in a recent overview of methods for estimating the TTSN (16).
As the concept of survival normalization has provided valuable insights into the prognosis of various NHLs in several recent studies, it is worth taking a deeper look into some of the potential limitations of the applied methods. From a statistical perspective, determining TTSN is complex as the number of patients at risk in a study becomes smaller with increasing follow-up and thereby weakening the power of statistical tests to detect a true difference between the patient and background population. Therefore, TTSN findings could be a result of underpowered statistical tests and researchers could falsely conclude survival normalization too early (Figure 1) (17). Instead of relying on statistical tests for detection of the TTSN, the LEL may be used to quantify the expected number of years lost due to a specific disease. This is computed by calculating the area between the patient and background population survival curves until a given time point, e.g., 5 or 10 years (Figure 2). This is often referred to as the restricted LEL, as it only provides the number of life years lost within a specific time window. Since the restricted LEL does not capture the impact of the disease on survival over a longer period, for example the full lifetime of an individual, this has prompted extrapolations of both the patient and background population survival curves beyond the observed follow-up to quantify the LEL over the full lifetime of patients (18,19). However, extrapolations are by nature prone to uncertainty as they rely on survival assumptions beyond the available data points and without possibility to confirm the accuracy of the applied assumptions. Extrapolation to estimate the total LEL has been tested within colon cancer, breast cancer, malignant melanoma, and bladder cancer with varying accuracy (18,20). Improved extrapolations may be obtained by using approaches that utilize data with long follow-up, such as population-based registers or background population mortality rate tables (21). Assumptions can then be based on the expected survival difference between the patients and the background population without need for assumptions directly on the patient survival. Nonetheless, the validity of the extrapolations remain untestable and there is no guarantee against biased extrapolations affecting the total LEL (18). Even if a model fits the data well within the observed follow-up, extrapolations based on the same model may perform poorly. Finally, the likelihood of large extrapolation biases increases if the expected residual lifetime of the patients is long, as seen in Hodgkin lymphoma patients (22,23), because extrapolation over a longer time period is required. Sudden changes in outcomes, for example due to a rise in the incidence of fatal late complications such as secondary cancers, may also lead to poor extrapolations.
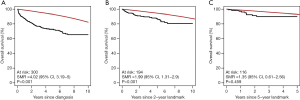
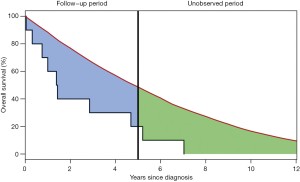
Another potential bias when comparing NHL patient survival to the survival of the background population is systematic selection of fit patients for treatment. This is particularly relevant when assessing elderly patients, as an elevated mortality beyond the identified TTSC may still exist if the NHL patient population is not matched to an identically fit background population, i.e., the differences between the patients and the background population is not limited to the presence of NHL. Additionally, frail NHL patients will be at increased risk of dying early during therapy due to complications or suboptimal treatment leading to poor disease control. Therefore, the patient population could be gradually enriched for a fitter patient segment over time which may lead to biased comparisons to a background population that has not been exposed to a similar selection process (24).
Survival normalization models for use in clinical practice
After the diagnosis of NHL, most patients engage actively in discussions with health care professionals about treatment options, possible treatment outcomes, and treatment toxicities. Many patients express a wish to know their prognosis which is typically assessed by using clinical prognostic scores such as the International Prognostic Index (IPI) and the FL International Prognostic Index (FLIPI) (25,26). The prognostic scores group the patients in 3–4 groups based on the number of risk factors present. The survival outcomes within each of the prognostic subgroups are then typically reported as 5-year OS estimates which in DLBCL ranges between >80% for low risk disease (IPI =0) and <40% for high risk disease (IPI >2) (27). While these measures are relevant for summarizing disease burden for groups of patients and facilitate comparisons of outcomes across institutions and clinical trials, they are not optimal for patient counselling. For example, a four-year OS estimate of 59% is detrimental information for a 20-year-old DLBCL patient with high-risk disease (IPI 4–5) due to poor Eastern Cooperative Oncology Group (ECOG) performance score caused by massive mediastinal bulk disease, extensive extranodal involvement, and elevated LDH. On the contrary, an 80-year-old with advanced stage disease, elevated lactate dehydrogenase and poor performance due to age-related comorbidities with similar IPI-predicted 4-year survival of 59% may find great comfort in this information. The same prognostic information can be perceived so differently because crude 5-year OS estimates do not account for the differences in expected residual lifetime between a 20-year-old and an 80-year-old patient. Another caveat of using 5-year OS for patient counselling is that 5-year OS estimates of less than 100% can be received with disappointment by patients for whom 100% OS may be synonymous with cure. Missing the opportunity to integrate normal life expectancy in the prognostic assessments can thus cause confusion. Finally, the use of prognostic scores calculated at diagnosis does not reflect the significant changes in prognosis as the patient reaches different milestones during treatment and follow-up.
Landmark analyses in DLBCL
The first study to analyze TTSN in DLBCL was an American-French study including 767 patients from the University of Iowa/Mayo Clinic Specialized Program of Research Excellence Molecular Epidemiology Resource (MER) and the North Central Cancer Treatment Group (NCCTG-N0489), all treated with immunochemotherapy and followed for a median of 5 years. The patient survival was compared to the survival of a matched background population and SMRs of observed to expected number of deaths were calculated at diagnosis for all patients and for patients reaching 12 and 24 months of event-free survival (EFS) (11). EFS was defined as the time from diagnosis until progression/relapse, unplanned NHL treatment, or death from any cause. At diagnosis, DLBCL patients had significantly increased risk of death as compared to the matched background population, which was reflected by an SMR of 2.88 (95% CI, 2.51 to 3.31). The SMR dropped to 1.40 (95% CI, 1.10 to 1.76) for patients reaching the EFS12 milestone, while the SMR was not significantly different from 1 (1.18, 95% CI, 0.57 to 1.57) for patients reaching the EFS24 milestone, suggesting normalized survival (11). The results were validated in a French cohort of 820 newly diagnosed DLBCL patients with a median follow-up of 42 months. Despite normalized survival at the EFS24 milestone, the DLBCL patients remained at risk of relapse with 8% of patients achieving EFS24 relapsing in the following 5 years. Thus, the TTSN does not imply true cure from NHL (11). The fact that relapses were not rare after EFS24, yet did not prevent survival normalization, supports that time from diagnosis to relapse is an important predictor of survival and that late relapses are generally more responsive to salvage therapies (28,29). Importantly, the IPI risk factors lost their prognostic value over time and was no longer predictive of outcome in patients reaching the EFS24 milestone. The results of this study were immediately applicable for patient counselling and spurred a widespread interest in TTSN milestones (12,22). After establishing EFS24 as an important milestone for DLBCL patients, the development of tools that predict the likelihood of achieving EFS24 was a natural next step to provide personalized prognostic information to DLBCL patients. Based on eight routinely collected clinical factors, a prediction model was developed in 1,348 patients treated with immunochemotherapy and successfully validated in an independent cohort of 1,177 patients (30). The model provides a personalized probability between 0% and 100% of reaching the EFS24 milestone and has improved discriminatory ability (C-statistic =0.671) over both IPI (C-statistic =0.649) and the National Comprehensive Cancer Network IPI (C-statistic =0.657).
The importance of reaching specific time points without events was later confirmed in nationwide population-based studies. A Danish Lymphoma Registry study investigated the TTSN in a cohort of DLBCL patients in complete remission (CR)/unconfirmed (CRu) after first-line R-CHOP-like treatment (12). Similarly to the American-French study, this study showed increased mortality after completion of first-line immunochemotherapy, despite all analyzed patients being in CR/CRu (SMR, 1.75, P<0.001). A small but significant excess mortality (SMR, 1.27, P<0.001) remained for patients in remission for 24 months and persisted even for patients in remission 48 months after therapy. However, the restricted LEL was substantially reduced after two years in remission. The small divergence between the US and Danish data may be explained by the nationwide scale of the Danish Lymphoma Registry, thereby reducing potential selection bias when comparing patient survival to that of the Danish background population. Notably, for patients <50 years of age, survival normalization was observed after 2 years possibly because the few late relapses in young DLBCL patients are likely to be cured with intensive salvage therapies and autologous stem cell transplantation. Consistent with the American-French results, IPI was no longer prognostic after 24 months. Both the French-American and Danish studies are limited by a relatively short follow-up, making extrapolation and full evaluation of LEL uncertain.
A more recent population-based study of LEL in DLBCL was based on the Swedish Lymphoma Registry (19). Unlike the two previous studies, this study examined trends over time in LEL and was not restricted to patients treated with R-CHOP(-like) therapies. Furthermore, OS time points after diagnosis, and not EFS or PFS time points like the two previous studies, were used to estimate potential survival normalization of DLBCL as data on DLBCL relapse were not available in the registry throughout the surveyed time period [2000–2013]. Rather than reporting SMRs, the Swedish study modelled LEL using flexible parametric survival models for all patients and for patients reaching the 2-year OS landmark. Extrapolations were used to compute the total LEL. Compared to the median age of 63 years in the MER cohort and 65 years in the Danish Lymphoma Registry study, the median age in the Swedish population-based study was 70 years, likely a result of not applying any restrictions on first-line chemotherapy. The study documented a decrease in LEL when comparing patients treated before and after the introduction of rituximab as standard therapy for DLBCL; LEL decreased from 8.0 to 4.6 years over the study period. The reduction in LEL was most pronounced for younger patients between 50 and 60 years and with age-adjusted IPI (aaIPI) ≥2 showing a decrease in LEL from 17.5 to 8.6 years for 50-year-old male patients from 2000 to 2013 and 18.4 to 9.1 years for female patients (19). For 50-year-old male patients with high risk disease reaching the two-year OS landmark in 2012, the LEL was no longer significantly increased (1.1 years, 95% CI, −2.3 to 4.5 years). For patients with aaIPI ≤2 and >2, LELs were reduced substantially from 3.8 and 6.1 years, respectively, at diagnosis to around 1 year for both groups after the two-year OS landmark. This study showed that the improvement in DLBCL outcomes over the surveyed time period was substantially stronger than the general increase in the life expectancy of the Swedish background population observed in the same era. OS landmarks are not directly comparable to PFS and EFS landmarks as patients who relapse before the 24 month landmark may reach the 2-year OS, but have a substantially increased risk of death compared to patients free of adverse events until the two-year landmark (11,12,19). Thus, for patient counselling and planning rational survivorship care, the PFS and EFS landmarks are more informative and provides a basis for more individualized risk assessment.
Landmark analyses in FL and marginal zone lymphoma (MZL)
Investigations of TTSN have also been performed in indolent NHLs. Casulo et al. evaluated the impact of progression of FL within two years of diagnosis in patients treated with first-line R-CHOP. The study demonstrated significantly reduced 5-year OS among patients with early progression (50%) compared to patients without events in the first two years (90%). In that study, survival was measured from the time of progression or from the two-year landmark in patients without progression (31). Normalization of OS in FL patients has previously been investigated using data from the American MER on 920 newly diagnosed FL patients and 412 FL patients from two French registries (32). The study revealed poor OS for patients with events within 12 months of diagnosis, whereas patients who remained event-free for 12 months did not have higher mortality rate than the background population (SMR, 0.73, 95% CI, 0.56–0.94). Among patients who initiated immunochemotherapy and remained event-free in the subsequent 24 months, the mortality was no longer elevated as compared to the background population (SMR, 0.37, 95% CI, 0.18–0.78).
An analysis of 263 Spanish FL patients undergoing rituximab containing treatment (median follow-up, 7 years) with validation in 693 patients from the GELTAMO group database (median follow-up, 6.7 years) explored similar research questions (33). This study investigated the clinical impact of being in complete response for 30 months after start of therapy (CR30), which corresponds to the time point for completion of induction therapy and 24 months of maintenance therapy. Among patients reaching the CR30 endpoint (71%), the 10-year relative survival (relative to the Spanish background population) was 100%, which was also seen in the GELTAMO validation cohort (62% reaching CR30), confirming normalization of OS in this subset of FL patients. Thus, CR30 appear to be a critical landmark in FL.
In other indolent lymphomas, such as extranodal MZL, unclassifiable low-grade B-cell lymphoma, and lymphoplasmacytic lymphoma, a recent American study by Tracy et al. compared the patient mortality with that of the US background population (34). Patients who were free of events (death, disease progression, and retreatment) 12 months after diagnosis experienced mortality rates similar to the background population (SMR 1.19, 95% CI, 0.95–1.46). On the contrary, patients with events within 12 months after diagnosis had significantly worse survival (SMR 3.13, 95% CI, 2.05–4.59) (34). A subsequent study exclusively evaluating the prognosis of MZL patients with planned immediate treatment after the diagnosis highlighted the excellent outcomes of MZL patients without adverse events within the first two years following diagnosis (35). For patients with disease progression within 24 months of diagnosis, the 3-year OS was 53%, whereas the survival of patients without progression within 24 months was 95%. However, because the treatment is generally not considered curable, the very positive outlooks for patients free of disease 24 months after diagnosis does not guarantee against future detrimental effects on survival due to multiple relapses over time.
Early time points for normalization of survival—ready for clinical trials?
While the majority of patients with DLBCL or FL respond to contemporary first-line immunochemotherapy, unmet medical needs exist for patients that are refractory or experience early relapse. For example, FL patients who relapse within the first 24 months after commencing first-line immunochemotherapy treatment have significantly worse OS than patients who achieve more durable remissions (5-year OS, 50% vs. 90%) (31,32). For DLBCL, first-line treatment has not improved since the addition of rituximab to CHOP in the early 2000s (36-38). Several attempts to improve the outcomes of DLBCL patients have failed, including the use of next generation CD20 antibodies, chemotherapy intensification with dose adjusted-EPOCH, and addition of small molecules that target active cellular pathways in the activated B-cell phenotype of DLBCL (39-43). However, a number of novel therapies are currently under evaluation in NHL such as bispecific antibodies directing T-cells towards CD20 positive cells (NCT03075696), genetically engineered T-cell therapies [chimeric antigen-receptor T-cell therapies (CART), ZUMA-7: NCT03391466], toxin conjugated antibodies (NCT03274492, NCT04150328), and macrophage activating anti-CD47 antibodies. These therapies are fundamentally different from the typical chemotherapy approach for relapsed/refractory NHL and while some are only tested in the relapsed/refractory setting, it is likely that they are relevant for high-risk DLBCL in the upfront setting as well. With the high number of drugs being screened for efficacy, it is critical to have reliable endpoints that accurately identifies drug candidates with a true benefit for the NHL patients.
SEPs are needed to bring new medicine faster to patients and to reduce the costs of clinical trials. In many cancer trials PFS and disease-free survival are used as preferred SEPs for OS due to their association with OS in combination with more events at earlier time points enabling faster readout. SEPs are not required to be as clinically meaningful as conventional endpoints, but changes in the SEPs should reflect changes in the conventional endpoint, i.e., the intervention effect based on the SEP accurately predicts the effect on the conventional endpoint (44,45). Correlation between the conventional endpoint and a new SEP is often intuitive or well documented, but despite a correlation, the effect of the clinical intervention measured with the SEP may be different from the effect on the conventional endpoint (44,46). Validating that the intervention effect based on the SEP predicts the effect on the conventional endpoint can be done by comparing the effect obtained on the two endpoints in a series of clinical trial data (47). For example, the hazard ratio (HR) is commonly used for measuring efficacy in cancer trials, and so the correlation between the HRs obtained by using OS and PFS as endpoints determines the validity of PFS as a SEP for OS. Validating the EFS24 milestone as SEP for OS would require a quantification of the correlation between the odds ratio (OR), or similar effect measures, for EFS24 and the HR for OS. As the concordance between the SEP and the conventional endpoint effects may be dependent on the therapeutic intervention under investigation, data from a large number of trials are needed to compute this correlation with a high confidence, which often precludes the needed rigorous validation of SEPs. Using validated SEPs in clinical trials is reasonable when correlation is strong. However, there is no consensus on the amount of correlation required to define SEPs as valid (46). Various methods for quantifying the correlation between the SEP and the conventional endpoint have been proposed, including simple correlation coefficients, coefficients of determination and variations hereof, and proportions of agreement (47). Recent lymphoma studies have mainly been focusing on the coefficients of determination (48,49).
While the TTSN may be used as SEP in future trials, it comes with a number of caveats. The use of, e.g., EFS24 as SEP would be based on the assumption that novel therapies for DLBCL do not alter the typical course of DLBCL and are not associated with severe late toxicities that substantially reduces life expectancy beyond the EFS24 endpoint. A therapeutic intervention could effectively achieve short remissions but be associated with serious and fatal late toxicities or change relapse kinetics, which could lead to erroneous conclusions when EFS24 is used as endpoint. Additionally, if the incremental efficacy of an intervention on EFS persists beyond the EFS24 milestone, using conventional EFS will be statistically more powerful than using the EFS24 endpoint. That is, the probability of detecting a true effect of the intervention is larger with EFS compared to EFS24 (11). On the other hand, if the full effect of an intervention occurs within the first 12 months, EFS24 is more powerful than the continuous EFS. Applying the EFS24 milestone as a SEP for OS in DLBCL trials requires only two years of follow-up for each patient in the trial. In comparison, some of the recent DLBCL trials have a median follow-up of 31.5–60 months (39,41,42). Besides accelerating trial readout, the EFS24 milestone also changes the analytical approach from a time-to-event outcome to a dichotomous endpoint, which avoids censoring issues, although loss to follow-up may still occur.
The appropriateness of using a PFS24 milestone as SEP for OS was recently investigated by correlating the OR using the PFS24 endpoint with the HR obtained by using the OS endpoint in a series of 13 clinical trials from the Surrogate Endpoints for Aggressive Lymphoma (SEAL) database conducted in the period 1998–2015 (49). The comparison led to a high correlation (R2≥0.75), but the correlation did not exceed the pre-defined correlation threshold of 0.8 (49). The lack of sufficient correlation between the PFS24 OR and the OS HR may be a result of the single time point focus of PFS24, whereas the OS HR is based on the entire follow-up. Neither changes in prognosis beyond PFS24 nor the timing of events before PFS24 are taken into account when PFS24 is the primary endpoint, and this may cause some discrepancy between trial results with PFS24 and OS as endpoint. Thus, correlation between PFS24 and OS results is still not sufficiently documented and the PFS24 milestone is not suitable for randomized trials in DLBCL at this time.
A similar analysis investigated if the CR30 endpoint could be used as SEP for PFS among previously untreated FL patients (48). The Follicular Lymphoma Analysis of Surrogate Hypothesis (FLASH) group analyzed patient-level data from 13 trials published after 1990 investigating either induction or maintenance therapy. The study revealed a high correlation between results using CR30 and PFS as endpoints, exceeding predefined correlation thresholds. It was concluded that the CR30 endpoint is an appropriate SEP for PFS in future trials of first-line FL therapy. As treatment for FL is typically not considered curative and new frontline treatment approaches may not necessarily increase OS due to availability of effective therapies after progression, improving CR30 is of important value for patients as long as it occurs in the absence of undue toxicities (50-52).
Conclusions
Understanding prognosis of NHL relative to the expected survival in an age and sex-matched background population is valuable for patients, health care planners, and potentially also for designing future clinical trials. Prognostic information delivered in an understandable language using measures that patients can directly relate to is important for active and informed engagement of patients and relatives in critical treatment decisions. The normalization of survival may also have a role as a SEP in future clinical trials to provide more rapid readout of study results compared to conventional endpoints such OS and PFS. However, from a statistical perspective, caution should be given when deriving new endpoints based on survival normalization because an excess mortality may persist beyond the point of survival normalization, especially if the number of patients with long follow-up is limited.
Acknowledgments
Funding: None.
Footnote
Reporting Checklist: The authors have completed the Narrative Review reporting checklist. Available at http://dx.doi.org/10.21037/aol-20-4
Conflicts of Interest: All authors have completed the ICMJE uniform disclosure form (available at http://dx.doi.org/10.21037/aol-20-4). LHJ received Honoraria from Takeda TCE and serves as an unpaid editorial board member of Annals of Lymphoma from Mar 2020 to Feb 2022; AKØ reports Travel expenses Pfizer (and Abbvie); MJM reports Research funding from Celgene/BMS and Nanostring, membership of Advisory Board for Morphosys, Pfizer, Kite Pharma, and serves as unpaid editorial board member of Annals of Lymphoma from Mar 2020 to Feb 2022; TCEG reports employment by Roche Ltd., and serves as an unpaid editorial board member of Annals of Lymphoma from Sep 2018 to Aug 2020. The other authors have no conflicts of interest to declare.
Ethical Statement: The authors are accountable for all aspects of the work in ensuring that questions related to the accuracy or integrity of any part of the work are appropriately investigated and resolved.
Open Access Statement: This is an Open Access article distributed in accordance with the Creative Commons Attribution-NonCommercial-NoDerivs 4.0 International License (CC BY-NC-ND 4.0), which permits the non-commercial replication and distribution of the article with the strict proviso that no changes or edits are made and the original work is properly cited (including links to both the formal publication through the relevant DOI and the license). See: https://creativecommons.org/licenses/by-nc-nd/4.0/.
References
- Friedberg JW. Relapsed/refractory diffuse large B-cell lymphoma. Hematology Am Soc Hematol Educ Program 2011;2011:498-505. [Crossref] [PubMed]
- Crump M, Neelapu SS, Farooq U, et al. Outcomes in refractory diffuse large B-cell lymphoma: results from the international SCHOLAR-1 study. Blood 2017;130:1800-8. [Crossref] [PubMed]
- Wästerlid T, Harrysson S, Andersson PO, et al. Outcome and determinants of failure to complete primary R-CHOP treatment for reasons other than non-response among patients with diffuse large B-cell lymphoma. Am J Hematol 2020;95:740-8. [Crossref] [PubMed]
- Shadman M, Li H, Rimsza L, et al. Continued Excellent Outcomes in Previously Untreated Patients With Follicular Lymphoma After Treatment With CHOP Plus Rituximab or CHOP Plus 131I-Tositumomab: Long-Term Follow-Up of Phase III Randomized Study SWOG-S0016. J Clin Oncol 2018;36:697-703. [Crossref] [PubMed]
- Sarkozy C, Maurer MJ, Link BK, et al. Cause of Death in Follicular Lymphoma in the First Decade of the Rituximab Era: A Pooled Analysis of French and US Cohorts. J Clin Oncol 2019;37:144-52. [Crossref] [PubMed]
- Bachy E, Seymour JF, Feugier P, et al. Sustained Progression-Free Survival Benefit of Rituximab Maintenance in Patients With Follicular Lymphoma: Long-Term Results of the PRIMA Study. J Clin Oncol 2019;37:2815-24. [Crossref] [PubMed]
- Johnson PWM, Rohatiner AZS, Whelan JS, et al. Patterns of survival in patients with recurrent follicular lymphoma: A 20-year study from a single center. J Clin Oncol 1995;13:140-7. [Crossref] [PubMed]
- Link BK, Day B, Zhou X, et al. Second-line and subsequent therapy and outcomes for follicular lymphoma in the United States: data from the observational National LymphoCare Study. Br J Haematol 2019;184:660-3. [Crossref] [PubMed]
- Tralongo P, Surbone A, Serraino D, et al. Major patterns of cancer cure: Clinical implications. Eur J Cancer Care (Engl) 2019;28:e13139 [Crossref] [PubMed]
- Andersson TM, Dickman PW, Eloranta S, et al. Estimating and modelling cure in population-based cancer studies within the framework of flexible parametric survival models. BMC Med Res Methodol 2011;11:96. [Crossref] [PubMed]
- Maurer MJ, Ghesquières H, Jais JP, et al. Event-free survival at 24 months is a robust end point for disease-related outcome in diffuse large B-cell lymphoma treated with immunochemotherapy. J Clin Oncol 2014;32:1066-73. [Crossref] [PubMed]
- Jakobsen LH, Bøgsted M, Brown P, et al. Minimal Loss of Lifetime for Patients With Diffuse Large B-Cell Lymphoma in Remission and Event Free 24 Months After Treatment: A Danish Population-Based Study. J Clin Oncol 2017;35:778-84. [Crossref] [PubMed]
- Boussari O, Romain G, Remontet L, et al. A new approach to estimate time-to-cure from cancer registries data. Cancer Epidemiol 2018;53:72-80. [Crossref] [PubMed]
- Romain G, Boussari O, Bossard N, et al. Time-to-cure and cure proportion in solid cancers in France. A population based study. Cancer Epidemiol 2019;60:93-101. [Crossref] [PubMed]
- Dal Maso L, Guzzinati S, Buzzoni C, et al. Long-term survival, prevalence, and cure of cancer: a population-based estimation for 818 902 Italian patients and 26 cancer types. Ann Oncol 2014;25:2251-60. [Crossref] [PubMed]
- Jakobsen LH, Andersson TML, Biccler JL, et al. On estimating the time to statistical cure. BMC Med Res Methodol 2020;20:71. [Crossref] [PubMed]
- Rabinowitz D, Ryan L. Lower Confidence Bounds for Time to Cure. Biometrika 1993;80:681. [Crossref]
- Andersson TML, Dickman PW, Eloranta S, et al. Estimating the loss in expectation of life due to cancer using flexible parametric survival models. Stat Med 2013;32:5286-300. [Crossref] [PubMed]
- Ekberg S, Jerkeman M, Andersson PO, et al. Long-term survival and loss in expectancy of life in a population-based cohort of 7114 patients with diffuse large B-cell lymphoma. Am J Hematol 2018;93:1020-8. [Crossref] [PubMed]
- Jakobsen LH, Andersson TML, Biccler JL, et al. Estimating the loss of lifetime function using flexible parametric relative survival models. BMC Med Res Methodol 2019;19:23. [Crossref] [PubMed]
- Jackson C, Stevens J, Ren S, et al. Extrapolating Survival from Randomized Trials Using External Data: A Review of Methods. Med Decis Making 2017;37:377-90. [Crossref] [PubMed]
- Hapgood G, Zheng Y, Sehn LH, et al. Evaluation of the Risk of Relapse in Classical Hodgkin Lymphoma at Event-Free Survival Time Points and Survival Comparison With the General Population in British Columbia. J Clin Oncol 2016;34:2493-500. [Crossref] [PubMed]
- Biccler JL, Glimelius I, Eloranta S, et al. Relapse Risk and Loss of Lifetime After Modern Combined Modality Treatment of Young Patients With Hodgkin Lymphoma: A Nordic Lymphoma Epidemiology Group Study. J Clin Oncol 2019;37:703-13. [Crossref] [PubMed]
- Hernán MA. The hazards of hazard ratios. Epidemiology 2010;21:13-5. [Crossref] [PubMed]
- The International Non-Hodgkin’s Lymphoma Prognostic Factors Project. A Predictive Model for Aggressive Non-Hodgkin’s Lymphoma. N Engl J Med 1993;329:987-94. [Crossref] [PubMed]
- Solal-Céligny P, Roy P, Colombat P, et al. Follicular Lymphoma International Prognostic Index. Blood 2004;104:1258-65. [Crossref] [PubMed]
- Biccler J, Eloranta S, de Nully Brown P, et al. Simplicity at the cost of predictive accuracy in diffuse large B-cell lymphoma: a critical assessment of the R-IPI, IPI, and NCCN-IPI. Cancer Med 2018;7:114-22. [Crossref] [PubMed]
- Farooq U, Maurer MJ, Thompson CA, et al. Clinical heterogeneity of diffuse large B cell lymphoma following failure of front-line immunochemotherapy. Br J Haematol 2017;179:50-60. [Crossref] [PubMed]
- Maurer MJ, Jakobsen LH, Schmitz N, et al. Age and Time to Progression Predict Overall Survival (OS) in Patients with Diffuse Large B-Cell Lymphoma (DLBCL) Who Progress Following Frontline Immunochemotherapy (IC). Blood 2019;134:400. [Crossref]
- Maurer MJ, Jais JP, Ghesquières H, et al. Personalized risk prediction for event-free survival at 24 months in patients with diffuse large B-cell lymphoma. Am J Hematol 2016;91:179-84. [Crossref] [PubMed]
- Casulo C, Byrtek M, Dawson KL, et al. Early relapse of follicular lymphoma after rituximab plus cyclophosphamide, doxorubicin, vincristine, and prednisone defines patients at high risk for death: An analysis from the National LymphoCare Study. J Clin Oncol 2015;33:2516-22. [Crossref] [PubMed]
- Maurer MJ, Bachy E, Ghesquières H, et al. Early event status informs subsequent outcome in newly diagnosed follicular lymphoma. Am J Hematol 2016;91:1096-101. [Crossref] [PubMed]
- Magnano L, Alonso-Alvarez S, Alcoceba M, et al. Life expectancy of follicular lymphoma patients in complete response at 30 months is similar to that of the Spanish general population. Br J Haematol 2019;185:480-91. [Crossref] [PubMed]
- Tracy SI, Larson MC, Feldman AL, et al. The utility of prognostic indices, early events, and histological subtypes on predicting outcomes in non-follicular indolent B-cell lymphomas. Am J Hematol 2019;94:658-66. [Crossref] [PubMed]
- Luminari S, Merli M, Rattotti S, et al. Early progression as a predictor of survival in marginal zone lymphomas: an analysis from the FIL-NF10 study. Blood 2019;134:798-801. [Crossref] [PubMed]
- Coiffier B, Lepage E, Brière J, et al. CHOP chemotherapy plus rituximab compared with CHOP alone in elderly patients with diffuse large-B-cell lymphoma. N Engl J Med 2002;346:235-42. [Crossref] [PubMed]
- Pfreundschuh M, Trümper L, Österborg A, et al. CHOP-like chemotherapy plus rituximab versus CHOP-like chemotherapy alone in young patients with good-prognosis diffuse large-B-cell lymphoma: a randomised controlled trial by the MabThera International Trial (MInT) Group. Lancet Oncol 2006;7:379-91. [Crossref] [PubMed]
- Habermann TM, Weller EA, Morrison VA, et al. Rituximab-CHOP versus CHOP alone or with maintenance rituximab in older patients with diffuse large B-cell lymphoma. J Clin Oncol 2006;24:3121-7. [Crossref] [PubMed]
- Younes A, Sehn LH, Johnson P, et al. Randomized Phase III Trial of Ibrutinib and Rituximab Plus Cyclophosphamide, Doxorubicin, Vincristine, and Prednisone in Non-Germinal Center B-Cell Diffuse Large B-Cell Lymphoma. J Clin Oncol 2019;37:1285-95. [Crossref] [PubMed]
- Vitolo U, Witzig TE, Gascoyne RD, et al. ROBUST: First report of phase III randomized study of lenalidomide/R-CHOP (R 2 -CHOP) vs placebo/R-CHOP in previously untreated ABC-type diffuse large B-cell lymphoma. Hematol Oncol 2019;37:36-7. [Crossref]
- Leonard JP, Kolibaba K, Reeves JA, et al. Randomized Phase 2 Open-Label Study of R-CHOP ± Bortezomib in Patients (Pts) with Untreated Non-Germinal Center B-Cell-like (Non-GCB) Subtype Diffuse Large Cell Lymphoma (DLBCL): Results from the Pyramid Trial (NCT00931918). Blood 2015;126:811. [Crossref]
- Bartlett NL, Wilson WH, Jung SH, et al. Dose-Adjusted EPOCH-R Compared With R-CHOP as Frontline Therapy for Diffuse Large B-Cell Lymphoma: Clinical Outcomes of the Phase III Intergroup Trial Alliance/CALGB 50303. J Clin Oncol 2019;37:1790-9. [Crossref] [PubMed]
- Vitolo U, Trněný M, Belada D, et al. Obinutuzumab or Rituximab Plus Cyclophosphamide, Doxorubicin, Vincristine, and Prednisone in Previously Untreated Diffuse Large B-Cell Lymphoma. J Clin Oncol 2017;35:3529-37. [Crossref] [PubMed]
- Fleming TR, Powers JH. Biomarkers and surrogate endpoints in clinical trials. Stat Med 2012;31:2973-84. [Crossref] [PubMed]
- Prentice RL. Surrogate endpoints in clinical trials: Definition and operational criteria. Stat Med 1989;8:431-40. [Crossref] [PubMed]
- Burzykowski T, Molenberghs G, Buyse M. The validation of surrogate end points by using data from randomized clinical trials: A case-study in advanced colorectal cancer. J Royal Stat Soc 2004;167:103-24. [Crossref]
- Sargent DJ, Wieand HS, Haller DG, et al. Disease-Free Survival Versus Overall Survival As a Primary End Point for Adjuvant Colon Cancer Studies: Individual Patient Data From 20,898 Patients on 18 Randomized Trials. J Clin Oncol 2005;23:8664-70. [Crossref] [PubMed]
- Shi Q, Flowers CR, Hiddemann W, et al. Thirty-month complete response as a surrogate end point in first-line follicular lymphoma therapy: An individual patient-level analysis of multiple randomized trials. J Clin Oncol 2017;35:552-60. [Crossref] [PubMed]
- Shi Q, Schmitz N, Ou FS, et al. Progression-Free Survival as a Surrogate End Point for Overall Survival in First-Line Diffuse Large B-Cell Lymphoma: An Individual Patient-Level Analysis of Multiple Randomized Trials (SEAL). J Clin Oncol 2018;36:2593-602. [Crossref] [PubMed]
- Salles G, Seymour JF, Offner F, et al. Rituximab maintenance for 2 years in patients with high tumour burden follicular lymphoma responding to rituximab plus chemotherapy (PRIMA): A phase 3, randomised controlled trial. Lancet 2011;377:42-51. [Crossref] [PubMed]
- Marcus R, Davies A, Ando K, et al. Obinutuzumab for the first-line treatment of follicular lymphoma. N Engl J Med 2017;377:1331-44. [Crossref] [PubMed]
- Morschhauser F, Fowler NH, Feugier P, et al. Rituximab plus lenalidomide in advanced untreated follicular lymphoma. N Engl J Med 2018;379:934-47. [Crossref] [PubMed]
Cite this article as: Jakobsen LH, Øvlisen AK, Eloranta S, Bøgsted M, Severinsen MT, Smedby KE, Maurer MJ, El-Galaly TC. A narrative review of survival normalization in non-Hodgkin lymphoma: useful for better patient counselling and an endpoint for clinical trials? Ann Lymphoma 2020;4:5.